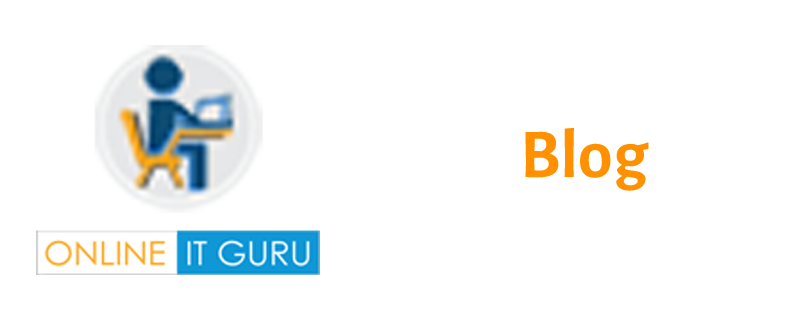
Hadoop is a distributed File System for processing large amount of data in a distributed Environment. Even the data is processed simultaneously there are some limitations. Let us know the draw backs of HDFS.
Limitations of Hadoop 1.0:
As both resource management and job progress need to be tracked, the maximum size of cluster is limited to 4000 nodes and the number of concurrent task is around 40000
HDFS has single point of failure, i.e If job tracker failed all queued and running jobs would be killed.
To overcome this problem Hadoop 2.0 was introduced.
YARN (YET ANOTHER RESOURCE NEGOTIATOR)/ HADOOP 2.0:
The scalability of resource allocation problem has resolved through dedicated resource Scheduler –YARN. It is a specific component of open source platform for big data analytics. It is also defined as a software rewrite which decouples the Map Reduce resource Management .and scheduling capabilities from the data processing component. This resource manager has no responsibility for running or monitoring job process. It does not care about the type of process running. It simply assigns the resources to the running jobs and provides a back up resources through Resource Manager Component to avoid single point of Failure.
The major concept involves in YARN is that, it allocates the resources to both general purpose and application specific components. In YARN, the application submission client submits the resources to the Resource manager. The Resource manager then allocates the resources to the particular application in order to prioritize the task and maintain big data analytics systems. Yarn also extends the power of Hadoop in data center for taking the advantage of linear scale storage, cost effective and processing. The major advantage of Hadoop 2.0 multiple Map Reduce versions can run at the same time. And more over these applications does not require JAVA.
Know more at Big Data Hadoop online training
Architecture: The architecture of Yarn shown below. Let’s discuss each component in detail.
Resource Manager: This component is responsible for allocating the resources to the cluster. It starts the cluster initially and allocates the resources and reallocates the cluster in case of Failure.
It has two main components:
Scheduler: As the name indicates, it is responsible only for allocating the resources to the application. It does not do any monitoring task and it does not guarantee for the failure of job either through software or hard ware.
Application Manager: It manages the applications in the cluster .It is responsible for the maintenance of application in the cluster. It is responsible for the application masters and restarting them in case of failure.
Node Manager: It executed on each computing node. It starts and monitors the containers assigned to is as well as the usage of resources. As a matter of fact It manages the user process on that machine.
Application master: This is responsible for running applications in the Hadoop cluster An application a master runs per application It negotiates the resources from the resource manager and works with the node manager.
Container : In the process of allocating resources to the applications , the resource manager has extensive information over application needs for better scheduling decisions across all applications in the cluster. This leads to resource request and the result called container .
Features:
Cluster Utilization: Generally Static map reduce utilizes the dynamic allocation of cluster resources.
Scalability: Especially As data processing power is increasing continuously, YARN’s Resource Manager continuously focuses on scheduling and keeps pace as clusters for managing the peta bytes of data.
Multi – tenancy : For simultaneous access of same data set , YARN allows multiple access engines to use Hadoop as a common standard for interactive , batch and real – time engines .
Compatibility: YARN is very compactable; it can run the already worked applications developed for Map Reduce 1 without any Distribution.
Recommended Audience :
Software developers ETL developersProject ManagersTeam Lead’sBusiness AnalystPrerequisites:
There is nothing much prerequisite for learning Big Data Hadoop .It’s good to have a knowledge on some OOPs Concepts . But it is not mandatory .Our Trainers will teach you if you don’t have a knowledge on those OOPs Concepts
Become a Master in HBase from OnlineITGuru Experts through Big Data Hadoop online course