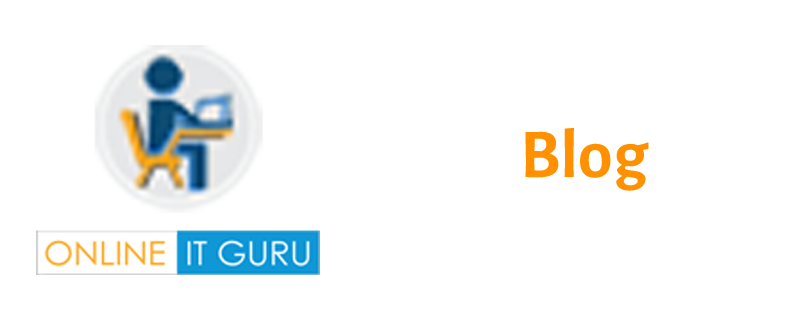
Developing Data science is different from software Development. Earlier the methods which are used for developing, software does not work for it. We can do software development with 100 % assurance. This will expect the output of it. Where Data exploration with a hypothesis, testing and learning will discover more outcomes. In the first place, this explains that is Developing data science is easy or a tough job.
Is developing Data science is easy:-
Developing Data science projects in comparison with a Software differs here.
- Reworking process
- Thinking what is good enough.
- Get knowledge on Art of failure.
- Data Discovery.
- Hypothesis Development.
- We should have Data.
Big Data:-
Generally, we should have Data, if it is Big data it is more than enough. Big Data is the answer to What is Data science. Big data has 3 dimensions known as volume, variety, and velocity. That refers to the amount of data, variety refers to many types of data, and velocity prefers the speed of data processing. The continuous growth of big data is not less. So then we can see tech watches, like apple and Fit bit. For the most part that made up of the Internet of things like photos, images and many more.
Hypothesis and Development Process:-
For instance, Data scientist Development process starts with a data scientist and Data science tutorial. He will recognize and predict the process of testing. This is the output of communicating with many business people. To understand the main sources Including business, variations. Brainstorming Data and variables will get a good result in performance.
Especially Data science Development method, requires the best communication. Business stakeholders on the complete development process. Afterwards Think and measure the source of finance operations, and users’ value designs. Get Ideas on the variables and calculations that give the best prediction of business performances.
Data Discovery:-
If you want to become the best data scientist, you be like the best detective. Ask many questions that design many statements. Which do many Discoveries and Investigations? A good data scientist quickly know what is a challenging sample.
Consequently, Data scientist methodology is that where, data scientist get a same or Interesting Data. Where data scientist will define and design the plan. By this, they will get the hypothesis that testing and know what, data sources are going to design Analytic samples. Furthermore, data scientist has a method that, gives the data visualization tools, to explore the data and, not show the correlations. Data engineering plays the main role in r for data science engagement process. It develops and designs large-scale data processing systems.
Get knowledge on Art of failure:-
The correct variables and metrics are not known in the before. The data science course encourages speed testing and learning. we can observe this process in data scientist credo. Simply Data science is about recognizing metrics and variables which will recognize the performance.
The process of data scientist development methodology, is real where statistics for data science work starts. Similarly Data scientist uses tools like sensor flow and many more to design analytic samples.
In this case best and most critical aspect of data science. Development is software developers should think that data science, work is not completed. They won't get 100 % accuracy in their work. We cannot see the clean point in data science work. In fact, analytics samples will go, constantly redefine on many changing factors like wear and tear, metal fatigue, local economics and weather designs.
Hold the learning and returning process:-
There it also considers in the best and predictable way. The continuous effectiveness of the analytical sample, monitored regularly or retrained regularly. The world will change constantly according to the economic conditions, and technology innovations. In the meantime, Data science Development has some genuine requirements. Integrate analytics process with the software development process.
Conclusion:-
Soon data science vs data analytics goes into the main concept, of many organizations. Lastly, Business stakeholders and product development have to think, that developing and operating analytical samples is different from, developing software. A good Data science team should be ready for whatever work is assigned to them and adjust accordingly.