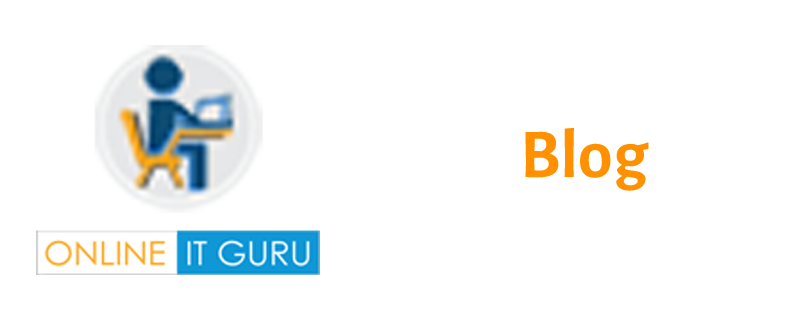
Due to the high usage of the internet, the amount of data generation is increasing day today. And this data gets generated in huge amounts in different formats. Hence we need to utilize the Big data concept to get the actual meaningful data for further utilization of data for experts. Moreover, today there are various methods and prospects to utilize this data. Hence today, in this article I'm going to explain various big data methods as well as the prospects.
Before going to know about the Big data methods and prospects, let us initially know
Why Big data is needed?
As mentioned above, the massive growth of data scaling in recent years is the key factor of this big data. Some experts define this big data in terms of 4V's. Those are Volume, varieties, Velocities, Veracities. And each of these terms requires high-performance processing. Hence addressing this big data is a challenging and time taking task. This requires large computation power to ensure successful data processing and analysis. So there is a requirement of data processing methods to process this huge data. And now we will through all those.
1)Data processing:
The set of techniques, utilized prior to the process of data mining is referred to as data preprocessing. And this is considered as the most meaningful issues with the famous knowledge discovery. Since the data will be imperfect, data inconsistencies and data redundancies will not directly affect the Data mining stating process. Moreover, today we have fast-growing of data generation rates and their sizes in business, industrial as well as the academic and the science applications. Besides, this huge amount of data require a more sophisticated mechanism to analyze it. But today Data pre-processing is able to adapt these requirements posting by each data mining algorithm. Besides, it enables to process the unfeasible data.
Get practical explanation of data processing at Big data Online TrainingHow Data preprocessing is done?
Albeit data processing is a perfect tool, that enables users to treat and process the complex data. Besides, it consumes a lot of processing time. Hence this includes a wide range of disciplines. And this varies due to the various data processing as well as the data preparation techniques. This further includes the data transformation, integration, cleaning as well as the normalization. And this aims to reduce the data complexity by the feature selection. Hence after the application of successful data preprocessing stage, the final data that obtained is regarded as the reliable and suitable source to any applied data mining algorithms.
Moreover, this Big data preprocessing is not limited to the task of data mining. Today more and more novel researchers in the novel data mining tasks are seriously increasing attention to the data preprocessing. And the Analyst will consider this as a tool to improve their models. Till now, I hope, you people have got enough idea regarding Big Data preprocessing. In the next paragraphs, we will be discussing more on these preprocessing techniques.
Data Preprocessing techniques:
There are various techniques to deal with data preprocessing. And we will discuss some of them
Imperfect data:
Most of the data mining techniques rely on data that is noise-free. In real-time, the data is from free (or) complete. In data processing, it is common to employ the techniques to remove the nosing data (or) to fill the missing data. Hence the imperfect data is further classified into the following two types:
a)Missing values imputation:
One of the major mistake that data analyst assume is that data is complete. But the presence of missing values is very common in the acquisition values. Moreover, the missing value is the datum that is not stored(or) gathered during the faulty sampling process, cost reductions (or) the limitations in the acquisition process. Moreover, the treatment of the missing values is different. And analyst must perform this with atmost care. Any mistake done during this process will lead to false conclusions. Besides many analysts today report that these missing values cause inefficiency in the knowledge extraction process. Hence today many approaches were available to tackle the problem with the missing values in the data preprocessing.
The first option is to discard those instance that contains the missing value. But according to most of the analyst, this approach is not suggestable. This because eliminating the instances, may generate the bias in the leaning process. And also predict that any important information may be deleted. And the analyst model the probable data functions to take into account which induces the missingness. Hence with the utilization of likelihood procedures analyst sample the appropriate probabilistic models to fill the missing values. Since the probability model for the particular data set is unknown analyst make use of machine learning techniques. And these Machine learning techniques were very useful in these scenarios without any prior information.
b)Noise treatment:
Most of the analyst assumes that the data they received is noiseless. Moreover, in most of the cases, this data gathering is rarely perfect and the corruptions may often appear. Since the data mining technique is dependent on data quality, tackling the noise data problem is mandatory. Hence in case of supervised problems, noise can affect the input features/ output features (or) both. And when the noise present in the input attribute, it is known as the attribute noise. And the second refer to the noise filters. This identifies and removes the noise data in the training data set.
2)Dimensionality reduction:
When the data set becomes large in the number of predictor variables, (or) number of instances, data mining algorithms will face the curse of dimensionality problems. The analyst considers this as a serious problem which obstructs the big data mining algorithms w.r.t the computation cost rise. And this dimension reduction can happen in the following ways
a)Feature Selections:
This the process of identifying and removing the redundant as well as useless data as much as possible. Its goal is to obtain the subset of features from the original problem that appropriately describe it. This subset is responsible to train the learner. This adds benefits in the specialized feature. Moreover, this Big data technique can induce accidental co-relations in the learning algorithms. Besides, this also reduces the algorithm overfitting. Moreover, this also reduces the number of dimensions
b)space transformations:
Besides the selection of most promising features, these big data techniques generate the new data features by combining with the original ones. And analyst can done this using any different criteria. The initial approaches were based on linear methods. This includes factor analysis as well as PCA
c)Instance reduction
This is the popular approach to minimize the impact of large data sets. It reduces the size the dataset without any decrease of quality of the knowledge. And it is a complementary task regarding FS. It reduces the data quantity by removing the instances (or) generating new ones.
d)Instance selection:
This is considered as the necessary ones in the process of data analysis. The main problem here is to identify suitable examples. And the analyst identifies these examples from the large number of instances. Moreover, this instance selection comprises of series of techniques. And you can select the data subset that replaces the original subset. And a successful Instance selection will produce the minimum data set. Moreover, this data set is independent of data mining algorithms
e)Instance generation:
This method is a contrast to instance selection. Besides the data selection, this Instance generation can generate and replaces the new data with the original data. And this process all the analyst to fill the domain problem. And these methods were often known as prototype generation methods.
Hence likewise, there are many methods and techniques to process this big data. And I hope you people have got the basic stuff of these techniques. And you can get more live techniques by OnlineITGuru live experts at Big data training.