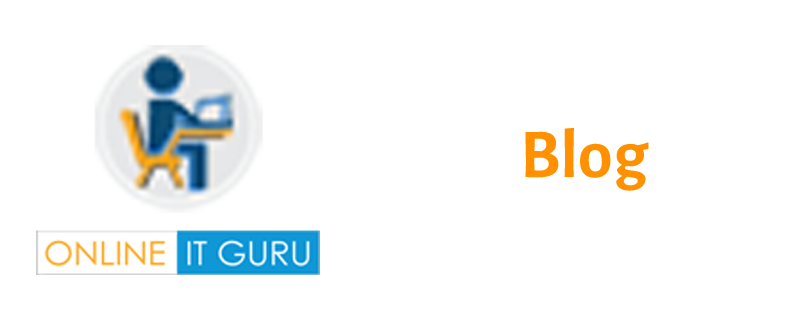
Data Cleaning
Data scientists spend most of their time in Cleaning data. For this they don't need any innovative ideas. Data cleaning is critical because, if they get any error it results in non-quality results. This will effect the decision making process that based on results. In this blog 5 simple steps for data cleaning will provide you best tips. With this tips any data scientist or analyst implement data cleaning in their company.
https://www.youtube.com/watch?v=rsMarPYG9xAChecking Correlation
In my point of view, it is unethical considering statistical tools in data science. We have one reason, that non-parametric analytics, either these are free from spreading of parameters, or distributed with certain specified parameters. Not to mention I would keep the mutual results easily, before going to implement any model. So that I will be having easy sense of data and future results. Moreover I can find a best method to use as same, while non-predictive analysis and big correlations that show effects on modeling features. We have algorithm for data cleaning.
Enroll for Data science IT Certifications training
It is not an issue, if you use R or Python, or any other language. We have many options to operate these checks. When they do work with big data. It always should have a minimum speed, for small subset of data for many different methods for dealing tests. This will guide you to save total time taken to operate your code. This explains how to clean big data.
Implementing Descriptive checks
Descriptive checks are well-known and they are useful in checking and defining. How many number of categories are there. I need to keep analysis or create sense to combine certain categories as only one. This type of decisions are mainly performed in a way that sample counts for every category. This is the best indicator for selecting a preferred category in regression analyses. Many machine learning algorithms work well to deal with non-stable Instance sizes in the category variable. Not to mention by looking a the Data, pre-processing category variables will help in improving results. This process includes data cleaning steps in data mining.
Recognizing Time Differences
Do or Do Not, I am predicting and Viewing Time series Data. One of the best and first things, I look when am working with any temporary data. It can assumed as, it is variation in outcome variable of every time period. For any type, time series prediction, it is mandatory and it must be mixed in predictions. And they have way of direction from the starting and it always being considered as advantageous. However, even it is for result driven analytics, the season ability that show, how you make up the data to get exact results. It is done by Data cleaning strategies.
Detecting and maintaining outliers
It looks it is clear, I noticed when I am correcting few analysis at work. That outputs modifying dramatically, when the outliers terminated. I won’t go into certain specific points, but the outliers become much important in the present analytics and predictions. By looking at visual of analytics, outliers looked differently. As a matter of fact it always very useful to update and keep, in mind while going with results. It Guides machine learning approach to data cleaning.
Work with one single and unique check
A perfect check is useful for understanding, the design of data and make sure that. There is no fake observations in data. For Instance, if a user purchase, many products from a store every day, it is must to assume, that daily data will be unique, at the level of user product. However, if we see a product among many products, they get them by Store, that seen the same. But it is available in many different colors. The data will be genuine, at the level of customer product, instead of our starting Assumption of a user-product level. Tracking for uniqueness before mixing the data, it is also very useful; by this, we can terminate fake values. These are best data cleaning steps in data science. As I mentioned, it does n’t require any updated techniques. Data science with Python is so simple.
The above all are the best steps about cleaning Data. Data and Data science are big concepts but we can learn them easily. So Data cleaning is the most important part, every data scientist must know about data cleaning process.
OnlineITGuru is the best Data science online course provider. If you are interested in data science training, enroll with onlineITGuru. It give training on both, big data and data science concepts.